Contact

+49 9131 85 27826

+49 9131 85 27270
Address
Universität Erlangen-Nürnberg
Chair of Computer Science 5 (Pattern Recognition)
Martensstraße 3 91058 Erlangen Germany
Powered by
|
Mario Amrehn M. Sc.
Alumnus of the Pattern Recognition Lab of the Friedrich-Alexander-Universität Erlangen-Nürnberg
Towards an optimized workflow in interventional medicine.
A Semi-Automated Usability Evaluation Framework for Interactive Image Segmentation Systems Mario Amrehn, Stefan Steidl, Reinier Kortekaas, Maddalena Strumia, Markus Weingarten, Markus Kowarschik, Andreas Maier
-
For complex segmentation tasks, the achievable accuracy of fully automated systems is inherently limited. Specifically, when a precise segmentation result is desired for a small amount of given data sets, semi-automatic methods exhibit a clear benefit for the user. The optimization of human computer interaction (HCI) is an essential part of interactive image segmentation. Nevertheless, publications introducing novel interactive segmentation systems (ISS) often lack an objective comparison of HCI aspects. It is demonstrated that even when the underlying segmentation algorithm is the same throughout interactive prototypes, their user experience may vary substantially. As a result, users prefer simple interfaces as well as a considerable degree of freedom to control each iterative step of the segmentation.
In this article, an objective method for the comparison of ISS is proposed, based on extensive user studies. A summative qualitative content analysis is conducted via abstraction of visual and verbal feedback given by the participants. A direct assessment of the segmentation system is executed by the users via the system usability scale (SUS) and AttrakDiff-2 questionnaires. Furthermore, an approximation of the findings regarding usability aspects in those studies is introduced, conducted solely from the system-measurable user actions during their usage of interactive segmentation prototypes. The prediction of all questionnaire results has an average relative error of 8.9%, which is close to the expected precision of the questionnaire results themselves. This automated evaluation scheme may significantly reduce the resources necessary to investigate each variation of a prototype’s user interface (UI) features and segmentation methodologies.
Interactive Neural Network Robot User Investigation for Medical Image Segmentation Mario Amrehn, Maddalena Strumia, Markus Kowarschik, Andreas Maier
-
Interactive image segmentation bears the advantage of correctional updates to the current segmentation mask when compared to fully automated systems. Especially in the field of inter-operative medical image processing of a single patient, where a high accuracy is an uncompromisable necessity, a human operator guiding a system towards an optimal segmentation result is a time-efficient constellation benefiting the patient.
There are recent categories of neural networks which can incorporate human-computer interaction (HCI) data as additional input for segmentation. In this work, we simulate this HCI data during training with state-of-the-art user models, also called robot users, which aim to act similar to real users given interactive image segmentation tasks.
We analyze the influence of chosen robot users, which mimic different types of users and scribble patterns, on the segmentation quality. We conclude that networks trained with robot users with the most spread out seeding patterns generalize well during inference with other robot users.
Bildverarbeitung für die Medizin 2019 (BVM) (Bildverarbeitung für die Medizin (BVM)), Lübeck, 18.03.2019, pp. 56-61, 2019, ISBN 978-3-658-25325-7 (BiBTeX, Who cited this?)
Ideal Seed Point Location Approximation for GrowCut Interactive Image Segmentation Mario Amrehn, Maddalena Strumia, Stefan Steidl, Tim Horz, Markus Kowarschik, Andreas Maier
-
The C-arm CT X-ray acquisition process is a common modality in medical imaging. After image formation, anatomical structures can be extracted via segmentation. Interactive segmentation methods bear the advantage of a dynamically adjustable trade-off between time and achieved segmentation quality for the object of interest w. r. t. fully automated approaches. The segmentation’s quality can be measured in terms of the Dice coefficient with the ground truth segmentation image. A user’s interaction traditionally consist of drawing pictorial hints on an overlay image to the acquired image data via a graphical user interface (UI). The quality of a segmentation utilizing a set of drawn seeds varies depending on the location of the seed points in the image. In this paper, we (1) investigate the influence of seed point location on segmentation quality and (2) propose an approximation framework for ideal seed placements utilizing an extension of the well established GrowCut segmentation algorithm and (3) introduce a user interface for the utilization of the suggested seed point locations. An extensive evaluation of the predictive power of seed importance is conducted from hepatic lesion input images. As a result, our approach suggests seed points with a median of 72.5% of the ideal seed points’ associated Dice scores, which is an increase of 8.4% points to sampling the seed location at random. | 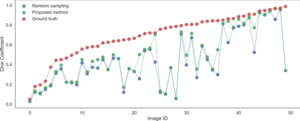
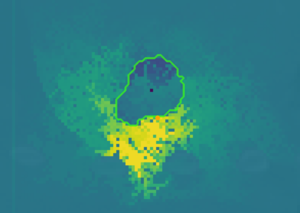
|
Bildverarbeitung für die Medizin 2018 (BVM), Erlangen, 11.03.2018, pp. 210-215, 2018, ISBN 978-3-662-56536-0 (BiBTeX, Who cited this?)
Robust Seed Mask Generation for Interactive Image Segmentation Mario Amrehn, Stefan Steidl, Markus Kowarschik, Andreas Maier
-
In interactive medical image segmentation, anatomical structures are extracted from reconstructed volumetric images. The first iterations of user interaction traditionally consist of drawing pictorial hints as an initial estimate of the object to extract. Only after this time consuming first phase, the efficient selective refinement of current segmentation results begins. Erroneously labeled seeds, especially near the border of the object, are challenging to detect and replace for a human and may substantially impact the overall segmentation quality. We propose an automatic seeding pipeline as well as a configuration based on saliency recognition, in order to skip the time-consuming initial interaction phase during segmentation. A median Dice score of 68.22% is reached before the first user interaction on the test data set with an error rate in seeding of only 0.088%. | 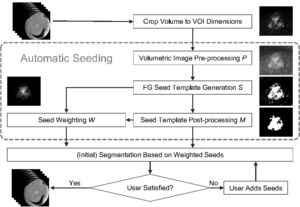
|
2017 IEEE Nuclear Science Symposium and Medical Imaging Conference Record (NSS/MIC) (IEEE Nuclear Science Symposium and Medical Imaging Conference (NSS/MIC)), Atlanta, Georgia, USA, 21.10-28.10.2017, pp. tbd, 2017 (BiBTeX, Who cited this?)
UI-Net: Interactive Artificial Neural Networks for Iterative Image Segmentation Based on a User Model Mario Amrehn, Sven Gaube, Mathias Unberath, Frank Schebesch, Tim Horz, Maddalena Strumia, Stefan Steidl, Markus Kowarschik, Andreas Maier
-
For complex segmentation tasks, fully automatic systems are inherently limited in their achievable accuracy for extracting relevant objects. Especially in cases where only few data sets need to be processed for a highly accurate result, semi-automatic segmentation techniques exhibit a clear benefit for the user. One area of application is medical image processing during an intervention for a single patient. We propose a learning-based cooperative segmentation approach which includes the computing entity as well as the user into the task. Our system builds upon a state-of-the-art fully convolutional artificial neural network (FCN) as well as a simple rule based active user model for training. During the segmentation process, a user of the trained system can iteratively add additional hints in form of pictorial scribbles as seed points into the FCN system to achieve an interactive and precise segmentation result. The segmentation quality of interactive FCNs is evaluated. Iterative FCN approaches can yield superior results compared to networks without the user input channel component, due to a consistent improvement in segmentation quality after each interaction. | 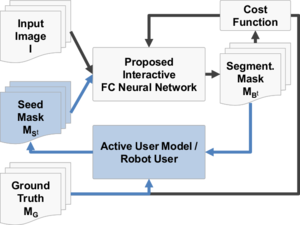
|
EG VCBM 2017 (Eurographics Workshop on Visual Computing for Biology and Medicine), Bremen, Germany, 2017-09-08, pp. 143-147, 2017, ISBN 978-3-03868-036-9 (BiBTeX, Who cited this?)
Comparative Evaluation of Interactive Segmentation Approaches Mario Amrehn, Jens Glasbrenner, Stefan Steidl, Andreas Maier
-
Image segmentation is a key technique in image processing with the goal to extract important objects from the image. This evaluation study focuses on the segmentation quality of three different interactive segmentation techniques, namely Region Growing, Watershed and the cellular automaton based GrowCut algorithm. Three different evaluation measures are computed to compare the segmentation quality of each algorithm: Rand Index, Mutual Information, and the Dice Coefficient. For the images in the publicly available ground truth data base utilized for the evaluation, the GrowCut method has a slight advantage over the other two. The presented results provide insight into the performance and the characteristics with respect to the image quality of each tested algorithm. | 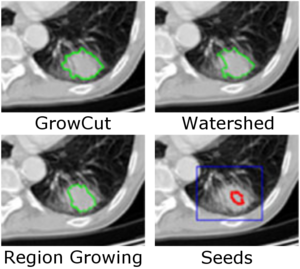
|
Bildverarbeitung für die Medizin 2016 (Bildverarbeitung für die Medizin), Charité - Universitätsmedizin Berlin, 13.03.2016, pp. 68-73, 2016, ISBN 978-3-662-49465-3 (BiBTeX, Who cited this?)
The Random Forest Classifier in WEKA: Discussion and New Developments for Imbalanced Data Mario Amrehn, Firas Mualla, Elli Angelopoulou, Stefan Steidl, Andreas Maier
-
Data analysis and machine learning have become an integrative part of the modern scientific methodology, providing automated techniques to predict further information based on observations. One of these classification and regression techniques is the random forest approach. Those decision tree based predictors are best known for their good computational performance and scalability.
However, in case of severely imbalanced training data, as often seen in medical studies' data with large control groups, the training algorithm or the sampling process has to be altered in order to improve the prediction quality for minority classes.
In this work, a balanced random forest approach for WEKA is proposed. Furthermore, the prediction quality of the unmodified random forest implementation and the new balanced random forest version for WEKA are evaluated against reference implementations in R. Two-class problems on balanced data sets and imbalanced medical studies' data are investigated. A superior prediction quality using the proposed method for imbalanced data is shown compared to the other three techniques.
Portability of TV-Regularized Reconstruction Parameters to Varying Data Sets Mario Amrehn, Andreas Maier, Frank Dennerlein, Joachim Hornegger
-
In C-arm computed tomography there are certain constraints due to the data acquisition process which can cause limited raw data. The reconstructed image’s quality may significantly decrease depending on these constraints. To compensate for severely under-sampled projection data during reconstruction, special algorithms have to be utilized, more robust to such ill-posed problems. In the past few years it has been shown that reconstruction algorithms based on the theory of compressed sensing are able to handle incomplete data sets quite well. In this paper, the iterative iTV reconstruction method by Ludwig Ritschl et al. is analyzed regarding it’s elimination capabilities of image artifacts caused by incomplete raw data with respect to the settings of it’s various parameters. The evaluation of iTV and the data dependency of iterative reconstruction’s parameters is conducted in two stages. First, projection data with severe angular under-sampling is acquired using an analytical phantom. Proper reconstruction parameters are selected by analyzing the reconstruction results from a set of proposed parameters. In a second step multiple phantom data sets are acquired with limited angle geometry and a small number of projections. The iTV reconstructions of these data sets are compared to short-scan FDK and SART reconstruction results, highlighting the distinct data dependence of the iTV reconstruction parameters. | 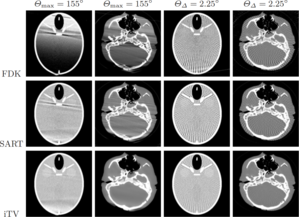
|
Bildverarbeitung fuer die Medizin 2016 (Workshop Bildverarbeitung fuer die Medizin), Berlin, 13.3.2016, pp. 277-282, 2016 (BiBTeX, Who cited this?)
Proceedings of the 2016 IEEE International Symposium on Biomedical Imaging: From Nano to Macro (2016 IEEE 13th International Symposium on Biomedical Imaging (ISBI)), Prague, 13.4.2016, pp. 589-592, 2016 (BiBTeX, Who cited this?)
Bildverarbeitung für die Medizin 2015, Lübeck, 16.03.2015, pp. 131-136, 2015, ISBN 978-3-662-46223-2 (BiBTeX, Who cited this?)
Transcatheter Arterial Chemoembolization (TACE)
-
This project aims at semi-automatic detection and segmentation of focal liver lesions in volumetric C-arm CT images. CT images are commonly used for diagnosis, treatment planning and follow-up examinations of liver tumors. The C-arm CT images are acquired during the curative intervention after intravenous application of a contrast agent.
To detect and segment the target lesions simultaneously, a voxel classification approach is pursued. During pre-processing, the search space is confined by segmenting the liver first.
Primary liver tumors are among the most frequent malignant tumors. More than 600,000 people worldwide die of hepatic cancer every year, according to the annual reports of the American Cancer Society. Especially colon cancer patients have a high risk of developing liver metastases at some point. However, so far computer assistance for physicians during diagnosis and surgical treatment of liver tumors is usually very basic.
This project aims at overcoming this deficit by providing an semi-automatic segmentation method feasible to use during an intervention. Given a set of CT images, it provides guidance for an accurate segmentation of liver lesions. The tumors' outline information is used to identify extra-hepatic collateral vessels feeding the cancerous tissue with oxigenated blood. Additionally, with an exact segmentation available, tumors can be monitored over a longer period of time in order to allow an assessment of tumor growth or shrinkage and thus the success of the treatment.
A major contribution of this work will be the highly accurate and fast semi-automatic segmentation of liver lesions. In particular, we want to focus on inhomogeneous. Current semi-automatic segmentation methods, which are often based on thresholding, usually benefit heavily from well-shaped, homogenous tumors, but fail when given irregular cases. Therefore, machine learning techniques will be applied to robustly detect and segment these tumors in images.
TACE is a minimally Invasive treatment for liver cancer. During TACE, a catheter is inserted into the femoral artery and subsequently navigated to the patient's vessels feeding the tumor with oxygenated blood.
In order to successfully occlude all nourishing extra-hepatic collateral vessels (high efficacy) while preserving the surrounding healthy tissue (low toxicity), an exact segmentation of the hepatic tissue is crucial.
The aim of this project is to enable and support an ultra selective TACE approach during the intervention by providing a framework to interactively perform an accurate and fast lesion segmentation in the interventional environment.
|